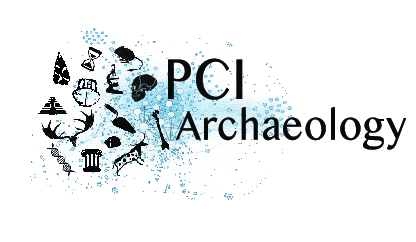
Assessing glutamine deamination in ancient parchment samples
Parchment Glutamine Index (PQI): A novel method to estimate glutamine deamidation levels in parchment collagen obtained from low-quality MALDI-TOF data
Abstract
Recommendation: posted 12 September 2022, validated 30 September 2022
Demarchi, B. (2022) Assessing glutamine deamination in ancient parchment samples. Peer Community in Archaeology, 100019. https://doi.org/10.24072/pci.archaeo.100019
Recommendation
Data authenticity and approaches to data authentication are crucial issues in ancient protein research. The advent of modern mass spectrometry has enabled the detection of traces of ancient biomolecules contained in fossils, including protein sequences. However, detecting proteins in ancient samples does not equate to demonstrating their endogenous nature: instead, if the mechanisms that drive protein preservation and degradation are understood, then the extent of protein diagenesis can be used for evaluating preservational quality, which in turn may be related to the authenticity of the protein data.
The post-mortem deamidation of asparaginyl and glutamyl residues is a key degradation reaction, which can be assessed effectively on the basis of mass spectrometry data, and which has accrued a long history of research, both in terms of describing the mechanisms governing the reactions and with regard to the best strategies for assessing and quantifying the extent of glutamine (Gln) and asparagine (Asn) deamidation in ancient samples (Pal Chowdhury et al., 2019; Ramsøe et al., 2021, 2020; Schroeter and Cleland, 2016; Simpson et al., 2016; Solazzo et al., 2014; Welker et al., 2016; Wilson et al., 2012).
In their paper, Nair and colleagues (2022) build on this wealth of knowledge and present a tool for quantifying the extent of Gln deamidation in parchment. Parchment is a collagen-based material which can yield extraordinary insights into manuscript manufacturing practices in the past, as well as on the daily lives of the people who assembled and used them (“biocodicology”) (Fiddyment et al., 2021, 2019, 2015; Teasdale et al., 2017). Importantly, the extent of deamidation can be directly related to the quality of the parchment produced: rapid direct deamidation of Gln is induced by the liming process, therefore high extents of deamidation are linked to prolonged exposure to the high pH conditions which are typical of liming, thus implying lower-quality parchment.
Nair et al.’s approach focuses on collagen peptides which are typically detected during MALDI-TOF mass spectrometry analyses of parchment and build a simple three-step workflow able to yield an overall index of deamidation for a sample (the parchment glutamine index - PQI) 一 taking into account that different Gln residues degrade at different rates according to their micro-chemical environment. The first step involves pre-processing the MALDI spectra, since Nair et al. are specifically interested in maximising information which can be obtained by low-quality data. The second step builds on well-established methods for quantifying Q → E from MALDI-TOF data by modelling the convoluted isotope distributions (Wilson et al., 2012). Once relative rates of deamidation in selected peptides within a given sample are calculated, the third step uses a mixed effects model to combine the individual deamidation estimates and to obtain an overall estimate of the deamidation for a parchment sample (PQI).
The PQI can be used effectively for assessing parchment quality, as the authors show for the dataset from Orval Abbey. However, PQI could also have wider applications to the study of processed collagen, which is widely used in the food and pharmaceutical industries. In general, the study by Nair et al. is a welcome addition to a growing body of research on protein diagenesis, which will ultimately improve models for the assessment of the authenticity of biomolecular data in archaeology.
References
Chowdhury, P.M., Wogelius, R., Manning, P.L., Metz, L., Slimak, L., and Buckley, M. 2019. Collagen deamidation in archaeological bone as an assessment for relative decay rates. Archaeometry 61:1382–1398. https://doi.org/10.1111/arcm.12492
Fiddyment, S., Goodison, N.J., Brenner, E., Signorello, S., Price, K., and Collins, M.J.. 2021. Girding the loins? Direct evidence of the use of a medieval parchment birthing girdle from biomolecular analysis. bioRxiv. https://doi.org/10.1098/rsos.202055
Fiddyment,S., Holsinger, B., Ruzzier, C., Devine, A., Binois, A., Albarella, U., Fischer, R., Nichols, E., Curtis, A., Cheese, E., Teasdale, M.D., Checkley-Scott, C., Milner, S.J., Rudy, K.M., Johnson, E.J., Vnouček, J., Garrison, M., McGrory, S., Bradley, D.G., and Collins, M.J. 2015. Animal origin of 13th-century uterine vellum revealed using noninvasive peptide fingerprinting. Proc Natl Acad Sci U S A 112:15066–15071. https://doi.org/10.1073/pnas.1512264112
Fiddyment, S., Teasdale, M.D., Vnouček, J., Lévêque, É., Binois, A., and Collins, M.J. 2019. So you want to do biocodicology? A field guide to the biological analysis of parchment. Heritage Science 7:35. https://doi.org/10.1186/s40494-019-0278-6
Nair, B., Rodríguez Palomo, I., Markussen, B., Wiuf, C., Fiddyment, S., and Collins, M. Parchment Glutamine Index (PQI): A novel method to estimate glutamine deamidation levels in parchment collagen obtained from low-quality MALDI-TOF data. BiorRxiv, 2022.03.13.483627, ver. 6 peer-reviewed and recommended by Peer community in Archaeology. https://doi.org/10.1101/2022.03.13.483627
Ramsøe, A., Crispin, M., Mackie, M., McGrath, K., Fischer, R., Demarchi, B., Collins, M.J., Hendy, J., and Speller, C. 2021. Assessing the degradation of ancient milk proteins through site-specific deamidation patterns. Sci Rep 11:7795. https://doi.org/10.1038/s41598-021-87125-x
Ramsøe, A., van Heekeren, V., Ponce, P., Fischer, R., Barnes, I., Speller, C., and Collins, M.J. 2020. DeamiDATE 1.0: Site-specific deamidation as a tool to assess authenticity of members of ancient proteomes. J Archaeol Sci 115:105080. https://doi.org/10.1016/j.jas.2020.105080
Schroeter, E.R., and Cleland, T.P. 2016. Glutamine deamidation: an indicator of antiquity, or preservational quality? Rapid Commun Mass Spectrom 30:251–255. https://doi.org/10.1002/rcm.7445
Simpson, J.P., Penkman, K.E.H., and Demarchi, B. 2016. The effects of demineralisation and sampling point variability on the measurement of glutamine deamidation in type I collagen extracted from bone. J Archaeol Sci 69: 29-38. https://doi.org/10.1016/j.jas.2016.02.002
Solazzo, C., Wilson, J., Dyer, J.M., Clerens, S., Plowman, J.E., von Holstein, I., Walton Rogers, P., Peacock, E.E., and Collins, M.J. 2014. Modeling deamidation in sheep α-keratin peptides and application to archeological wool textiles. Anal Chem 86:567–575. https://doi.org/10.1021/ac4026362
Teasdale, M.D., Fiddyment, S., Vnouček, J., Mattiangeli, V., Speller, C., Binois, A., Carver, M., Dand, C., Newfield, T.P., Webb, C.C., Bradley, D.G., and Collins M.J. 2017. The York Gospels: a 1000-year biological palimpsest. R Soc Open Sci 4:170988. https://doi.org/10.1098/rsos.170988
Welker, F., Soressi, M.A., Roussel, M., van Riemsdijk, I., Hublin, J.-J., and Collins, M.J. 2016. Variations in glutamine deamidation for a Châtelperronian bone assemblage as measured by peptide mass fingerprinting of collagen. STAR: Science & Technology of Archaeological Research 3:15–27. https://doi.org/10.1080/20548923.2016.1258825
Wilson, J., van Doorn, N.L., and Collins, M.J. 2012. Assessing the extent of bone degradation using glutamine deamidation in collagen. Anal Chem 84:9041–9048. https://doi.org/10.1021/ac301333t
The recommender in charge of the evaluation of the article and the reviewers declared that they have no conflict of interest (as defined in the code of conduct of PCI) with the authors or with the content of the article. The authors declared that they comply with the PCI rule of having no financial conflicts of interest in relation to the content of the article.
BNis funded by the European Union’s Horizon 2020 research and innovation programme under the Marie Skłodowska-Curie grant agreement No. 801199. At the time of producing this work, IRP, SF, and MC were funded by the European Union’s EU Framework Programme for Research and Innovation Horizon 2020 under GrantAgreementNo. 787282(B2C),andIRPiscurrentlyfundedbytheEuropeanUnion’sHorizon2020Research and Innovation Programme under the Marie Skłodowska-Curie grant agreement No 956410. BM is funded by the University of Copenhagen throughtheDataScienceLaboratory. CWisfundedbytheIndependentResearch Fund Denmark (grant number: 8021-00360B) and the University of Copenhagen through the Data+ initiative. SF and MC are funded by the European Union’s EU Framework Programme for Research and Innovation Horizon 2020 under Grant Agreement No. 787282(B2C). MCisalso supported by the Danish National Research Foundation (DNRF128). We thank Julie Wilson (JW) for helpful comments and advice.
Evaluation round #2
DOI or URL of the preprint: https://www.biorxiv.org/content/10.1101/2022.03.13.483627v3
Version of the preprint: v3
Author's Reply, 22 Aug 2022
We once again thank the recommender and reviewers for their comments and feedback that helped improve our manuscript. We deposited the new version of our manuscript with changes and corrections on bioRxiv. In addition, we attach our reply as a PDF.
We sincerely hope that the current version will be in accordance with the reviewer's expectations.
Best regards,
The authors
Decision by Beatrice Demarchi, posted 07 Aug 2022
Dear Authors
I have received some further comments from the reviewers. I will be happy to recommend this preprint as long as you respond to one of the reviewers concerns re the utility of the index and carefully check peptide nomenclature throughout (see below)
Best
BD
Reviewed by anonymous reviewer 1, 15 Jul 2022
The authors have addressed my concerns and I am happy to recommend this article.
Reviewed by anonymous reviewer 3, 26 Jul 2022
The authors made several improvements to the text which clarify the methods and results. The authors also corrected the names of several peptides, although errors remain in the images of Figure 2 and 4, as well as in the supplementary materials.
Researchers often face limitations in both availability of material and the quality of data that can be recovered from historical and archaeological materials and so I agree with the authors’ sentiment that it is important to have tools which can provide as much information as possible with the data that is available. I am still concerned, however, with the utility of the index when more than 50% of the data fall outside the theoretical range for the index. The authors explain this as a problem of accurate baseline correction, but I think it would be worth elaborating on this point in the discussion as to why this would/would not unduly impact the interpretation of the results.
Evaluation round #1
DOI or URL of the preprint: https://www.biorxiv.org/content/10.1101/2022.03.13.483627v6
Author's Reply, 05 Jul 2022
We thank the recommender and reviewers for their comments and feedback that helped improve our manuscript. We deposited the new version of our manuscript with changes and corrections on bioRxiv. We respond to the comments in a detailed point-by-point fashion in the attached file.
We sincerely hope that the current version will be in accordance with the reviewer's expectations.
Best regards,
The authors
Decision by Beatrice Demarchi, posted 19 May 2022
Dear Authors
I have now received three reviews for your preprint entitled “PARCHMENT GLUTAMINE INDEX (PQI): A NOVEL METHOD TO ESTIMATE GLUTAMINE DEAMIDATION LEVELS IN PARCHMENT COLLAGEN OBTAINED FROM LOW-QUALITY MALDI-TOF DATA”
I am pleased to say that the reviews are mostly positive and recognise the importance of the work, and I agree with them. The reviewers raise valid points on some aspects of the manuscript, mainly concerning the choice of not screening out poorest-quality data. I share such concerns: after all, poor quality data is poor quality data, and no amount of post-acquisition work can really change that (and I agree with the reviewers that most of the data in Fig 2 looks very poor). My suggestion is that the authors provide an explanation of the reasons why looking at poor-quality data (rather than re-analysing samples in order to acquire better spectra and/or discarding the dataset) is deemed so important as to warrant a whole new application for assessing Q → E.
The other main question I have is on the reproducibility of the analytical settings across samples: the manuscript does not provide information on how the dataset was obtained, i.e. sample extraction, preparation, instrument, mass range, suppression, matrix type/concentration, operator(s), number of analytical/biological replicates, laser intensity, number of shots, etc…Maybe I could not find the information, but in any case it should be in the main text.
Another issue I would want to see addressed is that a few years ago Simpson et al published a paper demonstrating that MALDI-TOF is not ideal for Q → E because it underestimates E in peptide mixtures containing the Q and E forms (https://doi.org/10.1002/rcm.8441). While this might not be crucial if comparing relative difference between samples, I would mention this bias and justify why you still want to use MALDI, i.e you want to compare data that’s already been acquired/costs etc.
Finally, can the authors explain how they are separating the signal of parchment quality from that of time/preservation histories? The samples come from the same site, but they will have different post-production “biographies”, which may explain some of the variability observed. Can the authors comment on this? I appreciate that the focus of the manuscript is on the PQI index, but section “Applications of PQI” is a bit generic - the ms would benefit from a more nuanced interpretation of the data.
A general suggestion: refer to J. Gross’s manual for doubts on mass spectrometry terminology https://link.springer.com/book/10.1007/978-3-319-54398-7
I would ask you to revise the manuscript within one month, according to the comments of the three reviewers and the general points I made above, and to submit the revised preprint, along with a detailed point-by-point response. I shall be happy to recommend it, pending suitable revision.
Looking forward to receiving your revised manuscript.
Kinds regards
Beatrice Demarchi (Bea)
Reviewed by anonymous reviewer 1, 15 Apr 2022
The manuscript describes a method to calculate the level of glutamine deamidation for several peptides by modelling the convoluted isotope distributions and combine the results to give an overall estimate of the deamidation for a parchment sample. The method is described as a simple three-step workflow, where the modelling occurs in the second and the third steps. The modelling of individual peptides uses weighted least squares regression which seems sensible for the limited data although the weights are calculated according to the noise level estimated from at most 3 observations. The details of the regression in this section somehow suggest more data than is available, possibly due to the matrix X (line 208). It could be worth linking back to the fact that n is at most 3 (sometimes just 1), and (according to Figure 2) m is at most 6 (should this be 5?). The notation is confusing as xij suggests the element in the ith row of the jth column in the matrix X, but in fact this is used to denote the model for the ith isotopic peak in the jth replicate. Perhaps the latter could be denoted yij. Also 𝛾 is of length k rather than k+1.
The third step of the process uses a mixed effects model to combine the individual deamidation estimates and the mathematical details are provided. My worry is that, for all the statistical theory, the results may not be meaningful if the data are poor. The authors perform residual analysis and apart from a few badly fitted values, seem to have obtained good results. However, these results check the fit of the model to the peaks extracted from (as the title advertises) low quality data. This depends entirely on the first step in the workflow which involves pre-processing the MALDI spectra and the only way to assess the performance here is from Figure 2. Of the 5 randomly chosen spectra, 4 appear to show only noise in all but the first peptide in Figure 2A. Maybe the much greater intensities for sample 67_19_2 make the other spectra look worse than they are. In any case, apart from this sample, Figure 2B shows just how few peaks were identified (assuming that the solid circles indicate identified peaks) and it is difficult to see why the peaks in other samples were identified. In the case of 59_I_4_2, the intensities are just too low to see anything yet peaks were identified for several peptides. Maybe the weighted (normalised) spectra should be shown instead? However, even for sample 67_19_2, the pre-processing for the last peptide does not look convincing. Are these typical spectra?
Reviewed by Maria Codlin, 16 May 2022
This paper presents a novel method for quantifying the deamidation of collagen peptides in parchment derived from animal skins. They present a well-written paper and a thorough, if dense, overview of the methods and results. The R-package and model developed here provide interesting new avenues to examine the treatment of animal skins in the past. I do not have the background to speak to the suitability of the statistical models developed here and will focus on the peptides.
Peptide naming should be checked against reviewed collagen sequences to ensure correct and consistent naming throughout the paper. As this paper follows Brown et al. (2020), the peptide positions start counting from the beginning of the collagen-specific three-letter pattern (G-X-Y). Thus COL1a2 756-789 and COL1a2 535-567 in this paper are both COL1a2 756-789 and COL1a1 9-42 should be 10-42. Peptide COL1A1 375 is also referred to at certain points as 376. The reference for Brown et al. (2020) is also missing from the reference list.
While I believe the methods touch on this topic, I feel this paper requires a clearer explanation of how missing peaks are dealt with at the replicate and sample levels. If these are being estimated, as the methods seem to suggest, what are the implications of this to the final PQI value and why is this preferred over removing replicates where peaks are missing? It seems that many of the issues discussed in the paper, such as the high proportion of PQI values above 1 and the deviation of tails on the qq plots could be alleviated by screening out poorer quality spectra or increasing the signal to noise ratio. Given these issues, it is unclear whether background noise or missing values may be conflated with the deamidation models in the paper.
One of the two masses of peptide 9-42 is suggested to be incorrectly identified due to different rates of deamidation (lines 318-322). From Table 3, it is unclear which value is being referred to as the relative rates are very close in number. In contrast COL1a2 756-789 and its variant, referred to as COL1a2 535-567 have different relative rates of deamidation. As these are variants of the same peptide, is this different important and if so, how might it impact interpretations about the use of goat skins? The finding that goat hides were more heavily limed is intriguing. However, given that goats have a different variant of peptide COL1a2 756-789, I would like to see evidence that goat parchment is more heavily deamidated across multiple peptides to rule out any effect of the different rates of deamidation across the two variants of COL1a2 756-789.
Assuming the statistical analysis is sound, this study will make a valuable contribution to parchment production studies and the development of novel applications of MALDI-TOF in cultural heritage contexts.
Download the reviewReviewed by anonymous reviewer 2, 27 Apr 2022
This is an interesting paper that looks at deamidation as a marker of parchment quality, and allows the comparison of deamidation levels with other variables such as species and thickness of parchment to gain insight into the parchment making process. It is interesting that this baseline correction appears to improve the s/n of some peaks, but not others. I think the paper could be improved by acknowledging the limitations of the background correction technique and if possible, to draw some conclusions as to why this works for some, but not other peaks, is it to do with the s/n for the peak in the raw data? I am unable to comment on the mathematical/statistical aspects as this is outside my area of expertise.
Some minor corrections are recommended.
Lines 116-119: I don’t think this is true? You have a charge in MS2 as well, you can only see charged product ions and the product ion spectrum would be used for confirming the peptide sequence. The reason you have overlapping distributions in MS1 is due to the resolution of the instrument not the fact is the parent ion. In the future, since you have identified clear biomarkers it would be interesting to make a targeted MS method to carry out absolute quantitation using SRM on your 8 peptides.
Line 183: A s/n ratio of 1.5 is very low. Usually this is set at 3 for identification and higher than this for quantitative analysis (around 10) and what you are trying to do here is semi-quantitative.
Line 318 and 322: You could de-novo this to confirm?
Line 365: I’m not sure if authors mean that the base line correction did not work for this peptide in the sense that even after baseline correction, the s/n was too low?
Line 308: Why would a high level of deamidation in a peptide result in higher background if the background is caused by chemical interference from the MALDI matrix?
Line 384: MADI-TOF and ZooMS aren’t interchangeable. One is describing the ionisation technique/mass analyser and one is an application of MS to a sample type. Maybe better to say something like in the field of bioarcheology the application of MALDI-TOF to ancient samples is commonly referred to as ZooMS.
Line 391: I wonder if this variation due to the poor quality spectra or biological variation?
Line 400: It would be very difficult, if not impossible to validate this to EMA or FDA regulations.
Figure 2: It’s interesting that that for some peptides you can see an improvement in signal to noise when you compare them with the spectra in A vs B, but some still look poor quality after the background correction. Do you see any patterns in peptides where the correction works well for and which is doesn’t? I suspect that rather than the ionisation type, it’s the amount of collagen you are extracting in terms of s/n. If you remove deamidation levels from poor s/n spectra do your error bars change?
Figure 6: I’m not familiar with what level of spread of deamidation values you would expect for these samples but the error bars here seem quite high.
General comments: m/z should be italicised throughout.
Download the review