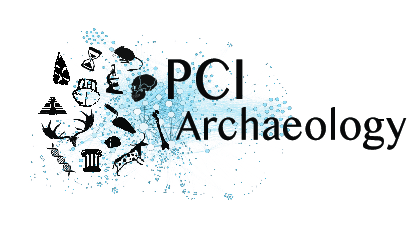
A Valuable Contribution to Archaeological Network Research: A Case Study of Pompeii
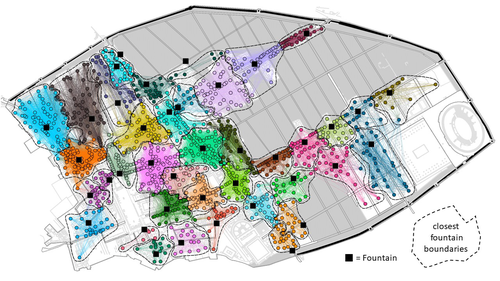
Social Network Analysis, Community Detection Algorithms, and Neighbourhood Identification in Pompeii
Abstract
Recommendation: posted 23 January 2024, validated 24 January 2024
Laguna-Palma, D. (2024) A Valuable Contribution to Archaeological Network Research: A Case Study of Pompeii. Peer Community in Archaeology, 100403. 10.24072/pci.archaeo.100403
Recommendation
The paper entitled 'Social Network Analysis, Community Detection Algorithms, and Neighbourhood Identification in Pompeii' [1] presents a significant contribution to the field of archaeological network research, particularly in the challenging task of identifying urban neighborhoods within the context of Pompeii. This study focuses on the relational dynamics within urban neighborhoods and examines their indistinct boundaries through advanced analytical methods. The methodology employed provides a comprehensive analysis of community detection, including the Louvain and Leiden algorithms, and introduces a novel Convex Hull of Admissible Modularity Partitions (CHAMP) algorithm. The incorporation of a network approach into this domain is both innovative and timely.
The potential impact of this research is substantial, offering new perspectives and analytical tools. This opens new avenues for understanding social structures in ancient urban settings, which can be applied to other archaeological contexts beyond Pompeii. Moreover, the manuscript is not only methodologically solid but also well-written and structured, making complex concepts accessible to a broad audience.
In conclusion, this study represents a valuable contribution to the field of archaeology, particularly for archaeological network research. Their results not only enhance our knowledge of Pompeii but also provide a robust framework for future studies in similar historical contexts. Therefore, this publication advances our understanding of social dynamics in historical urban environments. The rigorous analysis, combined with the innovative application of network algorithms, makes this study a noteworthy addition to the existing body of network science literature. It is recommended for a wide range of scholars interested in the intersection of archaeology, history, and network science.
Reference
[1] Notarian, Matthew. 2024. Social Network Analysis, Community Detection Algorithms, and Neighbourhood Identification in Pompeii. https://doi.org/10.5281/zenodo.8305968
The recommender in charge of the evaluation of the article and the reviewers declared that they have no conflict of interest (as defined in the code of conduct of PCI) with the authors or with the content of the article. The authors declared that they comply with the PCI rule of having no financial conflicts of interest in relation to the content of the article.
The authors declare that they have received no specific funding for this study
Evaluation round #1
DOI or URL of the preprint: https://doi.org/10.5281/zenodo.8305968
Version of the preprint: 2
Author's Reply, 15 Jan 2024
I sincerely appreciate the careful reviews returned here, especially the first reviewer who downloaded and tested the code and data. The reviews highlight a few important points regarding the clarity of the text and images, which I have responded to accordingly. I have detailed my specific responses below, in between the blockquotes. The tracked-changes version of the text should clarify how I revised the article.
Reviews
Reviewed by Matthew Peeples, 19 Oct 2023 03:54Overall, I found this to be a very clearly written and well documented methodological study with some quite useful new techniques. The dataset regarding doorways, buildings, paths, and fountains in Pompeii is truly awesome and there is so much potential here for urban network analyses. The approach to connecting neighborhoods/clusters in a network sense with neighborhoods in an archaeological urban environment is well developed and I particulary appreciate the nuanced discussion of nested areas around houses in terms of time and specific activities structuring mobility and social interactions at various scales. The general argument is very nicely illustrated with specific visual examples that work quite well.
I have very little to add to this paper as I thought it was very clear. The author is correct that the modularity based methods for defining network communities have rarely dug into the parameters involved in calculating modularity or the stability of solutions across different runs. I was only generally familiar with the CHAMP method prior to reading this piece and this shows a lot of potential. The reasoning behind selecting a range of modularity that produces stable community definitions actually makes more sense than simply maximizing modularity in many ways and I could see this approach being useful for a range of archaeological questions.
I really appreciate that this article presents this approach not as the sole solution to defining "correct" modularity ranges for defining communities but instead presents a set of heuristics to guide a researcher in the selection of an appropriate solution. For example, I appreciated that multiple solutions were investigated and compared to see how they related to each other but also that archaeological insights of the case at hand was used to inform the interpretation of the results (for example noting the problems with singleton neighborhoods and the close proximity among network communities in relation to specific features of the urban landscape like the forum). This is top notch exploratory data analysis which presents tools for developing an understanding of the underlying data rather than relying on strict rules and guidelines.
> Thank you!
The study is well documented in terms of code and data. I downloaded the data compendium and was able to reproduce the analyses on my own using the R code and Jupytr Notebook provided. The only hiccup was that parallel Leiden community detection doesn't work in a Windows environment apparently but the code proceeded to run in serial. I just had to wait a bit longer. I had to make some slight modifications in my own Python environment to run the code but the instructions were thorough so it wasn't difficult. The code notebook is well documented and the figures are for the most part quite readable (a little more on that below).
> I’m happy to learn that the code and data were accessible. Yes, the parallel issue is frustrating but beyond my expertise to fix.
I have just a few minor suggestions. First, the specific nature of how the travel time/path network used here was created and defined would be good. I would suggest adding a few sentences to describe the data and how they were converted into network objects and/or digitized. Further, I'd be interested to know how exactly paths between locations were determined in relation to buildings/entrances/presumed paths, etc. This is a minor thing but the current version doesn't really provide any detail.
> The methods relating to the creation of the spatial network were already published in the referenced paper, but I added a brief section describing it (lines 90-96). I also added a section describing the process used to convert the spatial network data into network objects (lines 108-119).
The last little quibble that I have is with regard to the colors used in figures 4, 6, and 8. The red and green together there are difficult for my particular color vision deficiency. I would recommend that you change the color palette of that figure. There is a useful colorblind color palette in the Seaborn package that works well for categorical data or color selection in complex figures.
> This is a fair criticism and I appreciate the reviewer pointing it out to me. Most of the colors used in these were the defaults output by the CHAMP python package. I changed these figures (now numbered 5, 7, and 9 after the addition of figure 4). I was able to edit the code to change the color scheme for the modularity mapping chart, which is now the default in my customized version of the CHAMP package. I used colors from the suggested Seaborn colorblind palette. I also ensured that each element has a unique shape or symbol so that colors are not needed to interpret the symbology. For example, I numbered the two partitions of interest so they can be referenced by number instead of color. I also used dotted lines and shading to indicate the relationship between the community stepped line and convex hull, so hopefully this clarifies some of the confusion expressed by reviewer 2.
> In order to reproduce the figures, I had to rerun the CHAMP algorithm as I did not have the raw data saved. Thankfully, the resulting top partitions were the same as when I had run the algorithm before writing this paper, underlining the validity of this approach. However, there were some minor differences in the number of “admissible” partitions returned, and these are reflected in the figures. I change some of the language in the text to reflect the most recent runs and align with the new figures.
Beyond this, I can think of a bunch of things I'd like to see happen with these data and the analyses presented here. For example, I think comparing specific partition overlaps using something like the Rand Index would be interesting to see which particular clusters are stable across different modularity definitions and in relation to stochastic processes. I would guess that the "belonging" measure used here would be closely related to these results and it would be exciting to see. I'd also love to hear more about the content of neighborhoods defined using different techniques and whether other kinds of infrastructure tends to fall along the same lines. This is, of course, well beyond the scope of this paper, but I'm excited to see what's next for these data.
> Thanks for the suggestions! I will certainly look into them as this study progresses.
Reviewed by Philip Verhagen, 20 Oct 2023 07:58
I found this a well written and thoughtful paper on a subject that so far has only seen limited application in archaeology. The CHAMP method has to my knowledge not been applied to any archaeological case, and seems to hold great promise for detecting communities in complex networks at multiple scales. The Pompeii case study clearly shows its added value for interpreting the spatial and social structures of its neighbourhoods.
The only part of the paper where I would like to see some changes is in the Methods section. Technical descriptions of how algorithms work are always very hard to understand when you haven’t actually used them, so pay some more attention to providing a description that is easier to understand for non-experts, in particular where it concerns introducing the core terminology which may be unfamiliar to many readers. Line 137-140, for example, introduces the concept of modularity without properly explaining it, and in line 140 the comment about it being NP complete is properly overcomplicating things for the purpose of this paper. Also, it will not be very clear to the reader why the stochastic results (line 143-144) are problematic, even when this is illustrated further down when showing the results of both algorithms.
> This is a fairly technical paper, so I would imagine the core readers would already have some background in network science. Nevertheless, I did add some sentences (lines 150-56) to clarify how the modularity algorithm works, and a brief note to preview the problem with stochastic results (lines 163-64). I disagree, however, that the mention of the NP completeness is overcomplicating. In my opinion, the sentence about NP completeness should be clear enough even without understanding the theoretical underpinnings of the term. Rather than overcomplicating the issue, I feel that this is a crucial point to understand why modularity does not produce the same answer every time you run an algorithm - it is too computationally complex to compute the absolute answer. Moreover, a curious reader could do a quick google search for the term, or simply skim the technical section if it is beyond their needs.
The explanation of the CHAMP method has similar issues. The concept of the convex hull does not really make sense to me without a proper illustration of how this works. Also, please explain what is displayed in the AMI heatmap. As a consequence, I found the accompanying graphs in Figures 4, 6 and 8 extremely difficult to interpret.
> I added a graph that illustrates how the convex hull is created for a given network (figure 4). I also added a section explaining what AMI is and how to interpret the heatmap (lines 222-25). The changes to these figures previously explained should make them easier to interpret.
When these issues are resolved, I wholeheartedly recommend this paper for publication.
> Thank you for your suggestions!
Reviewed by Isaac Ullah, 05 Oct 2023 16:32
This paper applies techniques from social network analysis to classify potential neighborhoods in the case study of Pompeii. Specificallyl, the Convex Hull of Admissible Modulatiry Partitions (CHAMP) algorithm is explored as a means to this end (and compared to other possible alogorithms). Using central public spaces -- in this case public fountains -- as "hubs" for the analysis, provides a good starting place that is potentially translatable to other case studies with some sort of public areas (courtyards, squares, plazas, etc.). Defining neighborhoods has been a major issue in houshold and urban archaeology, and many methods have been presented over the years, including space syntax, visibility, room block continuity, artifact refitting, stylistic analysis, and others. The approach employed in this paper leverages advances in social network analysis techniques (and software) to aid this type of delineation. By iteratively cycling through network thresholds to link houses to public fountains, a multi-scalar image of different possible neighborhood configurations emerges. I find this aspect to be particularly powerful, as often social phenomena occuring in urban centers would be nested in this way across different spatial scales.
I found the paper to be well written, reasonably concise, and easy to follow. The method is clearly explained, and could be easily replicated in another case study. The method is thus widely applicable, and appears to produce interesting and meangful results. A major benefit of this approach is that it provides multiple possible solutions that can be crosschecked with other data to expose the most reasonable configurations. This is more amenable to the realities of archaeological data in various settings, and, I think, is a more reasonable way to approach these types of problems. The paper presents multiple configurations for neighborhood configurations at Pompeii, although the main contribution of this work appears to be methodological. I recommend publishing with no major changes.
> Thank you very much!
Decision by David Laguna-Palma
, posted 15 Jan 2024, validated 17 Nov 2023
This manuscript represents a significant contribution to the field of archaeological network research, particularly in the challenging task of identifying urban neighborhoods within the context of Pompeii. The incorporation of network analysis into this domain is both innovative and timely. The paper is well-written and structured, with a particular focus on the relational dynamics within urban neighborhoods and the examination of their indistinct boundaries through advanced analytical methods. The manuscript provides a comprehensive analysis of community detection algorithms, including the Louvain and Leiden algorithms, and introduces a novel Convex Hull of Admissible Modularity Partitions (CHAMP) algorithm.
Nonetheless, based on the comments of the reviewers, I recommend that the author make minor revisions to the manuscript before it can be accepted for publication. The author should carefully consider the suggestions provided by the reviewers, which are attached below:
Reviewed by Matthew Peeples, 19 Oct 2023
Overall, I found this to be a very clearly written and well documented methodological study with some quite useful new techniques. The dataset regarding doorways, buildings, paths, and fountains in Pompeii is truly awesome and there is so much potential here for urban network analyses. The approach to connecting neighborhoods/clusters in a network sense with neighborhoods in an archaeological urban environment is well developed and I particulary appreciate the nuanced discussion of nested areas around houses in terms of time and specific activities structuring mobility and social interactions at various scales. The general argument is very nicely illustrated with specific visual examples that work quite well.
I have very little to add to this paper as I thought it was very clear. The author is correct that the modularity based methods for defining network communities have rarely dug into the parameters involved in calculating modularity or the stability of solutions across different runs. I was only generally familiar with the CHAMP method prior to reading this piece and this shows a lot of potential. The reasoning behind selecting a range of modularity that produces stable community definitions actually makes more sense than simply maximizing modularity in many ways and I could see this approach being useful for a range of archaeological questions.
I really appreciate that this article presents this approach not as the sole solution to defining "correct" modularity ranges for defining communities but instead presents a set of heuristics to guide a researcher in the selection of an appropriate solution. For example, I appreciated that multiple solutions were investigated and compared to see how they related to each other but also that archaeological insights of the case at hand was used to inform the interpretation of the results (for example noting the problems with singleton neighborhoods and the close proximity among network communities in relation to specific features of the urban landscape like the forum). This is top notch exploratory data analysis which presents tools for developing an understanding of the underlying data rather than relying on strict rules and guidelines.
The study is well documented in terms of code and data. I downloaded the data compendium and was able to reproduce the analyses on my own using the R code and Jupytr Notebook provided. The only hiccup was that parallel Leiden community detection doesn't work in a Windows environment apparently but the code proceeded to run in serial. I just had to wait a bit longer. I had to make some slight modifications in my own Python environment to run the code but the instructions were thorough so it wasn't difficult. The code notebook is well documented and the figures are for the most part quite readable (a little more on that below).
I have just a few minor suggestions. First, the specific nature of how the travel time/path network used here was created and defined would be good. I would suggest adding a few sentences to describe the data and how they were converted into network objects and/or digitized. Further, I'd be interested to know how exactly paths between locations were determined in relation to buildings/entrances/presumed paths, etc. This is a minor thing but the current version doesn't really provide any detail.
The last little quibble that I have is with regard to the colors used in figures 4, 6, and 8. The red and green together there are difficult for my particular color vision deficiency. I would recommend that you change the color palette of that figure. There is a useful colorblind color palette in the Seaborn package that works well for categorical data or color selection in complex figures.
Beyond this, I can think of a bunch of things I'd like to see happen with these data and the analyses presented here. For example, I think comparing specific partition overlaps using something like the Rand Index would be interesting to see which particular clusters are stable across different modularity definitions and in relation to stochastic processes. I would guess that the "belonging" measure used here would be closely related to these results and it would be exciting to see. I'd also love to hear more about the content of neighborhoods defined using different techniques and whether other kinds of infrastructure tends to fall along the same lines. This is, of course, well beyond the scope of this paper, but I'm excited to see what's next for these data.
Thanks,
Matt Peeples
Reviewed by Philip Verhagen, 20 Oct 2023
I found this a well written and thoughtful paper on a subject that so far has only seen limited application in archaeology. The CHAMP method has to my knowledge not been applied to any archaeological case, and seems to hold great promise for detecting communities in complex networks at multiple scales. The Pompeii case study clearly shows its added value for interpreting the spatial and social structures of its neighbourhoods.
The only part of the paper where I would like to see some changes is in the Methods section. Technical descriptions of how algorithms work are always very hard to understand when you haven’t actually used them, so pay some more attention to providing a description that is easier to understand for non-experts, in particular where it concerns introducing the core terminology which may be unfamiliar to many readers. Line 137-140, for example, introduces the concept of modularity without properly explaining it, and in line 140 the comment about it being NP complete is properly overcomplicating things for the purpose of this paper. Also, it will not be very clear to the reader why the stochastic results (line 143-144) are problematic, even when this is illustrated further down when showing the results of both algorithms.
The explanation of the CHAMP method has similar issues. The concept of the convex hull does not really make sense to me without a proper illustration of how this works. Also, please explain what is displayed in the AMI heatmap. As a consequence, I found the accompanying graphs in Figures 4, 6 and 8 extremely difficult to interpret.
When these issues are resolved, I wholeheartedly recommend this paper for publication.
Reviewed by Isaac Ullah, 05 Oct 2023
This paper applies techniques from social network analysis to classify potential neighborhoods in the case study of Pompeii. Specificallyl, the Convex Hull of Admissible Modulatiry Partitions (CHAMP) algorithm is explored as a means to this end (and compared to other possible alogorithms). Using central public spaces -- in this case public fountains -- as "hubs" for the analysis, provides a good starting place that is potentially translatable to other case studies with some sort of public areas (courtyards, squares, plazas, etc.). Defining neighborhoods has been a major issue in houshold and urban archaeology, and many methods have been presented over the years, including space syntax, visibility, room block continuity, artifact refitting, stylistic analysis, and others. The approach employed in this paper leverages advances in social network analysis techniques (and software) to aid this type of delineation. By iteratively cycling through network thresholds to link houses to public fountains, a multi-scalar image of different possible neighborhood configurations emerges. I find this aspect to be particularly powerful, as often social phenomena occuring in urban centers would be nested in this way across different spatial scales.
I found the paper to be well written, reasonably concise, and easy to follow. The method is clearly explained, and could be easily replicated in another case study. The method is thus widely applicable, and appears to produce interesting and meangful results. A major benefit of this approach is that it provides multiple possible solutions that can be crosschecked with other data to expose the most reasonable configurations. This is more amenable to the realities of archaeological data in various settings, and, I think, is a more reasonable way to approach these types of problems. The paper presents multiple configurations for neighborhood configurations at Pompeii, although the main contribution of this work appears to be methodological. I recommend publishing with no major changes.