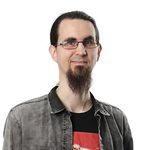
BRANDSEN Alex
- Digital Archaeology, Leiden University, Leiden, Netherlands
- Computational archaeology
- recommender
Recommendations: 3
Reviews: 2
Recommendations: 3
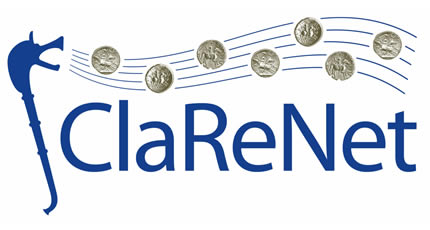
Supporting the analysis of a large coin hoard with AI-based methods
A demonstration of the use and finetuning of existing machine learning tools for analysing large complexes of coins
Recommended by Alex Brandsen based on reviews by 2 anonymous reviewersThe paper outlines the ClaReNet project's exploration of computer-based methods for classifying Celtic coin series, specifically focusing on a hoard from Jersey [1]. They collaborated with Jersey Heritage and numismatists, utilising a large dataset of coin images. The process involves stages such as pre-sorting, size-based sorting, class/type identification, and die studies. They employed IT methods, including object detection and unsupervised learning, followed by supervised learning for data refinement. Collaboration with numismatic experts ensured data quality. The study highlighted challenges in classifying coins, suggesting techniques like image matching alongside convolutional neural networks (CNNs). The results demonstrate the efficacy of semi-automatic processes in coin classification, emphasising the importance of human-computer collaboration for successful outcomes.
Overall, this is a good paper, showing how we as archaeologists and numismatics can use existing tools and finetune them for our purposes; without the need for huge domain specific datasets. This research and related papers show how we can more effectively deal with the increasingly bigger data we deal with, saving time on the monotonous and labour intensive tasks, leaving us more time to deal with the big picture. An important strength of the work is the provided public software repository and the dataset. The paper is well written, and a number of images illustrate the methodology as well as the objects used.
Reference
[1] Deligio, C., Tolle, K., and Wigg-Wolf, D. (2024). Supporting the analysis of a large coin hoard with AI-based methods. Zenodo, 8301464, ver. 4 peer-reviewed and recommended by Peer Community in Archaeology. https://doi.org/10.5281/zenodo.8301464
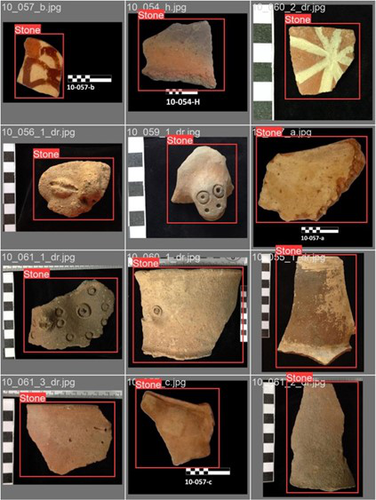
Machine Learning for UAV and Ground-Captured Imagery: Toward Standard Practices
A step forward in detecting small objects in UAV data for archaeological surveying
Recommended by Alex Brandsen based on reviews by 2 anonymous reviewersIn this paper [1], the authors describe how they apply machine learning with YOLOv5 to classify visual data, aiming to enhance understanding of archaeological phenomena before conducting destructive fieldwork. Despite challenges, the integration of machine learning with remote sensing technology was seen as transformative, enabling precise recording of areas of interest and assessment of environmental risk factors. The paper discusses successes, failures, and future directions in machine learning research, emphasising the need for standardisation and integration of streamlined methods. The application of machine learning techniques facilitates non-destructive analysis of material culture records, improving conservation efforts and offering insights into both past and contemporary phenomena. While the initial use of YOLOv5 showed potential for consistent detection of archaeological features, further refinement and dataset enlargement are deemed necessary for broader application in non-destructive archaeological surveying. The authors advocate for the integration of machine learning tools in archaeological research to save time, resources, and promote ethical digital recording practices. They highlight the importance of standardised methodologies to enhance credibility and reproducibility, aiming to contribute to the ongoing dialogue in computational archaeology.
Overall, I think this paper is a good step forward in detecting small objects in UAV data, and contains useful information for similar studies. The aim towards greater reproducibility and standardisation is of course shared more widely in the machine learning community, and this study is a good example of how to approach this.
References
[1] Sharp, K., Christofis, B., Eslamiat, H., Nepal, U. and Osores Mendives, C. (2024). Machine Learning for UAV and Ground-Captured Imagery: Toward Standard Practices. Zenodo, 8307612, ver. 5 peer-reviewed and recommended by Peer Community in Archaeology. https://doi.org/10.5281/zenodo.8307612
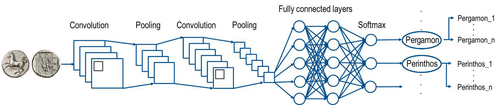
Creating an Additional Class Layer with Machine Learning to counter Overfitting in an Unbalanced Ancient Coin Dataset
A significant contribution to the problem of unbalanced data in machine learning research in archaeology
Recommended by Alex Brandsen based on reviews by Simon Carrignon, Joel Santos and 1 anonymous reviewerThis paper [1] presents an innovative approach to address the prevalent challenge of unbalanced datasets in coin type recognition, shifting the focus from coin class type recognition to coin mint recognition. Despite this shift, the issue of unbalanced data persists. To mitigate this, the authors introduce a method to split larger classes into smaller ones, integrating them into an 'additional class layer'.
Three distinct machine learning (ML) methodologies were employed to identify new possible classes, with one approach utilising unsupervised clustering alongside manual intervention, while the others leverage object detection, and Natural Language Processing (NLP) techniques. However, despite these efforts, overfitting remained a persistent issue, prompting the authors to explore alternative methods such as dataset improvement and Generative Adversarial Networks (GANs).
The paper contributes significantly to the intersection of ML techniques and archaeology, particularly in addressing overfitting challenges. Furthermore, the authors' candid acknowledgment of the limitations of their approaches serves as a valuable resource for researchers encountering similar obstacles.
This study stems from the D4N4 project, aimed at developing a machine learning-based coin recognition model for the extensive "Corpus Nummorum" dataset, comprising over 19,600 coin types and 49,000 coins from various ancient landscapes. Despite encountering challenges with overfitting due to the dataset's imbalance, the authors' exploration of multiple methodologies and transparent documentation of their limitations enriches the academic discourse and provides a foundation for future research in this field.
Reference
[1] Gampe, S. and Tolle, K. (2024). Creating an Additional Class Layer with Machine Learning to counter Overfitting in an Unbalanced Ancient Coin Dataset. Zenodo, 8298077, ver. 4 peer-reviewed and recommended by Peer Community in Archaeology. https://doi.org/10.5281/zenodo.8298077
Reviews: 2
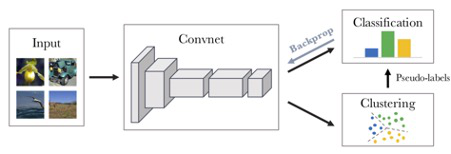
IT- and machine learning-based methods of classification: The cooperative project ClaReNet – Classification and Representation for Networks
Humans, machines, and the classification of Celtic coinage – the ClaReNet project
Recommended by Felix Riede, Sébastien Plutniak and Shumon Tobias Hussain based on reviews by Alex Brandsen and Eythan LevyThe paper entitled “IT- and machine learning-based methods of classification: The cooperative project ClaReNet – Classification and Representation for Networks” submitted by Chrisowalandis Deligio and colleagues presents the joint efforts of numismatists and data scientists in classifying a large corpus of Celtic coinage. Under the project banner “ClaReNet – Classification and Representation for Networks”, they seek to explore the possibilities and also the limits of new computational methods for classification and representation. This approach seeks to rigorously keep humans in the loop in that insights from Science and Technology Studies inform the way in which knowledge generation is monitored as part of this project. The paper was first developed for a special conference session convened at the EAA annual meeting in 2021 and is intended for an edited volume on the topic of typology, taxonomy, classification theory, and computational approaches in archaeology.
Deligio et al. (2024) begin with a brief and pertinent research-historical outline of Celtic coin studies with a specific focus on issues of classification. They raise interesting points about how variable degrees of standardisation in manufacture – industrial versus craft production, for instance – impact our ability to derive tidy typologies. The successes and failures of particular classificatory procedures and protocols can therefore help inform on the technological contexts of various object worlds and the particularities of human practices linked to their creation. These insights and discussion are eminently case-transferrable and applies not only to coinage, which after all is rather standardised, but to practically all material culture not made by machines – a point that gels particularly well with the contribution by Lucas (2022) to be published in the same volume. Although Deligio et al. do not flag this up specifically, the very expectation that archaeological finds should neatly fall into typological categories likely related to the objects that were initially used by pioneers of the method such as Montelius (1903) to elaborate its basic principles: these were metal objects often produced in moulds and very likely by only a very small number of highly-trained craftspeople such that the production of these objects approached the standardisation seen in industrial times (Nørgaard 2018) – see also Riede’s (2023) contribution due to be published in the same volume.
At the core of Deligio and colleagues’ contribution is the exploration of machine-aided classification that should, via automation, assist in handling the often large numbers of coins available in collections and, at times, from single sites or hoards. Specifically, the authors discuss the treatment of the remarkable coin hoard from Le Câtillon II on Jersey consisting of approximately 70,000 individual Celtic coins. They proceeded to employ several advanced supervised and unsupervised classification methods to process this stupendously large number of objects. Their contribution does not stop there, however, but also seeks to articulate the discussion of machine-aided classification with more theoretically informed perspectives on knowledge production. In line with similar approaches developed in-house at the Römisch-Germanische Kommission (Hofmann 2018; Hofmann et al. 2019; Auth et al. 2023), the authors also report on their cutely acronymed PANDA (Path Dependencies, Actor-Network and Digital Agency) methodology, which they deploy to reflect on the various actors and actants – humans, software, and hardware – that come together in the creation of this new body of knowledge. This latter impetus of the paper thus lifts the lid on the many intricate, idiosyncratic, and often quirky decisions and processes that characterise research in general and research that brings together humans and machines in particular – a concrete example of the messiness of knowledge production that commonly remains hidden behind the face of the published book or paper but which science studies have long pointed at as vital components of the scientific process itself (Latour and Woolgar 1979; Galison 1997; Shapin and Schaffer 2011). In this manner, the present contribution serves as inspiration to the many similar projects that are emerging right now in demonstrating just how vital a due integration of theory, epistemology, and method is as scholars are forging their path into a future where few if any archaeological projects do not include some element of machine-assistance.
References
Auth, Frederic, Katja Rösler, Wenke Domscheit, and Kerstin P. Hofmann. 2023. “From Paper to Byte: A Workshop Report on the Digital Transformation of Two Thing Editions.” Zenodo. https://doi.org/10.5281/zenodo.8214563
Deligio, Chrisowalandis, Caroline von Nicolai, Markus Möller, Katja Rösler, Julia Tietz, Robin Krause, Kerstin P. Hofmann, Karsten Tolle, and David Wigg-Wolf. 2024. “IT- and Machine Learning-Based Methods of Classification: The Cooperative Project ClaReNet – Classification and Representation for Networks.” Zenodo, ver.5 peer-reviewed and recommended by PCI Archaeology https://doi.org/10.5281/zenodo.7341342
Galison, Peter Louis. 1997. Image and Logic: A Material Culture of Microphysics. Chicago, IL: University of Chicago Press.
Hofmann, Kerstin P. 2018. “Dingidentitäten Und Objekttransformationen. Einige Überlegungen Zur Edition von Archäologischen Funden.” In Objektepistemologien. Zum Verhältnis von Dingen Und Wissen, edited by Markus Hilgert, Kerstin P. Hofmann, and Henrike Simon, 179–215. Berlin Studies of the Ancient World 59. Berlin: Edition Topoi. https://dx.doi.org/10.17171/3-59
Hofmann, Kerstin P., Susanne Grunwald, Franziska Lang, Ulrike Peter, Katja Rösler, Louise Rokohl, Stefan Schreiber, Karsten Tolle, and David Wigg-Wolf. 2019. “Ding-Editionen. Vom Archäologischen (Be-)Fund Übers Corpus Ins Netz.” E-Forschungsberichte des DAI 2019/2. E-Forschungsberichte Des DAI. Berlin: Deutsches Archäologisches Institut. https://publications.dainst.org/journals/efb/2236/6674
Latour, Bruno, and Steve Woolgar. 1979. Laboratory Life: The Social Construction of Scientific Facts. Laboratory Life : The Social Construction of Scientific Facts. Beverly Hills, CA: Sage.
Lucas, Gavin. 2022. “Archaeology, Typology and Machine Epistemology.” Zenodo. https://doi.org/10.5281/zenodo.7622162
Montelius, Gustaf Oscar Augustin. 1903. Die Typologische Methode. Stockholm: Almqvist and Wicksell.
Nørgaard, Heide Wrobel. 2018. Bronze Age Metalwork: Techniques and Traditions in the Nordic Bronze Age 1500-1100 BC. Oxford: Archaeopress Archaeology.
Riede, Felix. 2023. “The Role of Heritage Databases in Typological Reification: A Case Study from the Final Palaeolithic of Southern Scandinavia.” Zenodo. https://doi.org/10.5281/zenodo.8372671
Shapin, Steven, and Simon Schaffer. 2011. Leviathan and the Air-Pump: Hobbes, Boyle, and the Experimental Life. Princeton: Princeton University Press.
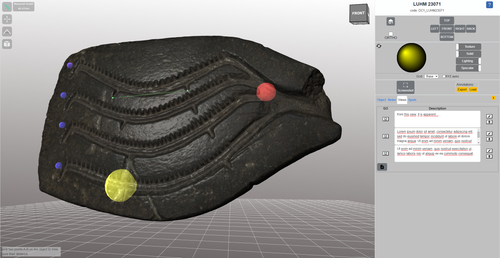
The Dynamic Collections – a 3D Web Platform of Archaeological Artefacts designed for Data Reuse and Deep Interaction
A comparative teaching and learning tool for 3D data: Dynamic Collections
Recommended by Sebastian Hageneuer based on reviews by Alex Brandsen and Louise TharandtThe paper (Callieri, M. et al. 2023) describes the “Dynamic Collections” project, an online platform initially created to showcase digital archaeological collections of Lund University. During a phase of testing by department members, new functionalities and artefacts were added resulting in an interactive platform adapted to university-level teaching and learning. The paper introduces into the topic and related works after which it starts to explain the project itself. The idea is to resemble the possibilities of interaction of non-digital collections in an online platform. Besides the objects themselves, the online platform offers annotations, measurement and other interactive tools based on the already known 3DHOP framework. With the possibility to create custom online collections a collaborative working/teaching environment can be created.
The already wide-spread use of the 3DHOP framework enabled the authors to develop some functionalities that could be used in the “Dynamic Collections” project. Also, current and future plans of the project are discussed and will include multiple 3D models for one object or permanent identifiers, which are both important additions to the system. The paper then continues to explain some of its further planned improvements, like comparisons and support for teaching, which will make the tool an important asset for future university-level education.
The paper in general is well-written and informative and introduces into the interactive tool, that is already available and working. It is very positive, that the authors rely on up-to-date methodologies in creating 3D online repositories and are in fact improving them by testing the tool in a teaching environment. They mention several times the alignment with upcoming EU efforts related to the European Collaborative Cloud for Cultural Heritage (ECCCH), which is anticipatory and far-sighted and adds to the longevity of the project. Comments of the reviewers were reasonably implemented and led to a clearer and more concise paper. I am very confident that this tool will find good use in heritage research and presentation as well as in university-level teaching and learning.
Although the authors never answer the introductory question explicitly (What characteristics should a virtual environment have in order to trigger dynamic interaction?), the paper gives the implicit answer by showing what the "Dynamic Collections" project has achieved and is able to achieve in the future.
Bibliography
Callieri, M., Berggren, Å., Dell'Unto, N., Derudas, P., Dininno, D., Ekengren, F., and Naponiello, G. (2023). The Dynamic Collections – a 3D Web Platform of Archaeological Artefacts designed for Data Reuse and Deep Interaction, Zenodo, 10067103, ver. 3 peer-reviewed and recommended by Peer Community in Archaeology. https://doi.org/10.5281/zenodo.10067103